Detecting Extreme Climate Event Impacts Using Remote Sensing Data in Deep Machine Learning Paradigms
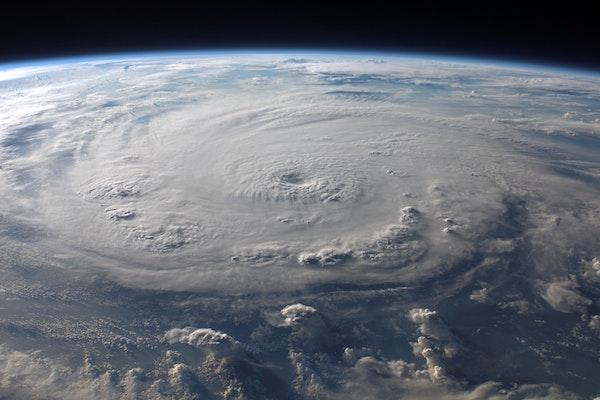
C.K. Shum, Distinguished University Scholar and Professor in the School of Earth Sciences (SES) at The Ohio State University and a Principal Investigator at the Byrd Center, co-authored a study presented at the American Geophysical Union's (AGU's) annual meeting last month in which his team, in collaboration with his international colleagues, used geodesy to study phenomena related to global climate change. Geodesy is the science of measuring the Earth, its geometry, and its spatial orientation.
Co-authors of the project were Yu Zhang, Yuanyuan Jia, Yihang Ding and Junyi Guo of Ohio State; Orhan Akyilmaz and Metehan Uz of Istanbul Technical University; and Kazim Atman of Queen Mary University of London.
By using remote sensing data from various space agency satellites in association with deep machine learning, the researchers created a system to monitor abrupt patterns in weather. The team produced several case studies to test their system to determine its accuracy in monitoring coastal sea-level changes before Hurricane landfalls by using reflected and collected radar signals from the International Global Navigation Satellite Systems (GNSS) satellite constellations by the GNSS receivers on the Earth's surface, or by the receivers on satellites orbiting the Earth in a much lower altitude, to monitor the evolution of landfalls from Hurricanes Harvey and Laura. GNSS provides "free" positioning, navigation, and timing services on a global or regional basis which are used for scientific research and commercial usage, generating billions of dollars in the US market in 2021. The use of the reflected and collected GNSS signals, which are all-weather, extends the applications of GNSS.
The team used over two-decade-long satellite gravimetry data collected by missions launched by the National Aeronautics and Space Administration (NASA) and the German Aerospace Center mission, GRACE, and its successor, GRACE Follow-On (GRACE-FO). Combining the gravimetry and other data with deep machine learning analytics, the team was able to reduce the resolution of the Earth's view from 600 km to 25 km and from monthly to daily re-visiting time, potentially improving the ability to monitor natural hazards. Preliminary study results include the monitoring of rapid evolutions of the 2017 Harvey and 2020 Laura Atlantic Hurricane landfalls,
"GRACE and GRACE Follow-On have been the unique technology during the last two decades that provide a capability to measure Earth's mass change, including climatic and abrupt weather episodes, which has become more frequent and more intense under an increasingly warmer Earth. However, their current limited temporal (monthly) and spatial resolution (660 km) is insufficient to fully detect the impact of extreme climate events, as such events occur in a shorter time (days to weeks) and over regions smaller than the GRACE's native spatial resolution." said Professor Orhan Akyilmaz, from Istanbul Technical University.
"Hydrometeorological processes largely govern the mass changes at a region. Here, we collaborated with the scientists at The Ohio States University and built a deep machine learning paradigm using training data, including the GRACE and GRACE-FO gravimetry observations and finer resolution hydrometeorological data from weather models, in situ data, and other remote sensing data, to "downscale" the original GRACE solutions. By this means which is ongoing, we were able to better localize the climate-induced hazard signal both in time and space." said Metehan Uz, a Ph.D. candidate from Istanbul Technical University, whose research is focused on the application of machine learning to study contemporary climate-induced hazards.
"The climate regimes worldwide are changing faster than ever, which is exposed by shifted seasons and higher occurrence frequency of extreme weather events. The power of deep machine learning models highly dependent on the number of data which they are able to learn from. Therefore, such models should be regularly updated with newly available data, in particular, for climatic-induced natural hazard studies." said Orhan Akyilmaz, Metehan Uz's Ph.D. research advisor.
"There are a number of challenges to possibly utilizing satellite gravimetry as a tool to monitor climate-induced natural hazards, including floods, droughts, storm surges, hurricanes, snow storms, etc. The challenges include the need to reduce the latency to acquire and process the satellite gravimetry data and methodologies to provide improved and timely solutions to generate the Earth's mass change observables for routine hazard monitoring. As the key training data in our research, improved satellite gravimetry solution is a requirement", said Yu Zhang, a postdoctoral fellow in SES at Ohio State.
The United States Agency supported this work for International Development (USAID), the National Science Foundation (NSF), NASA's Earth and Surface Interior Focus Area, and the Scientific and Technological Research Council of Türkiye (TÜBİTAK).
You can learn more by visiting Using machine learning to help monitor climate-induced hazards, as reported by Ohio State News.