New Hybrid Forecasting Approach Significantly Improves El Niño Predictions
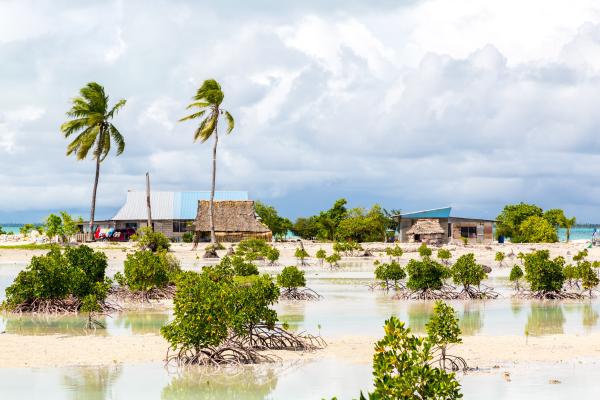
A recent international study published in Nature Communications has unveiled a new forecasting method that significantly enhances the prediction of El Niño–Southern Oscillation (ENSO) events, especially during the boreal spring, historically marked by diminished forecasting reliability. The strategy combines traditional dynamical climate models with deep learning methods.
The research, co-authored by The Ohio State University's Department of Geography Max Thomas Professor of Climate Dynamics, and Byrd Center Principal Investigator Zhengyu Liu, addresses a long-standing challenge in climate science: the accurate prediction of ENSO, a phenomenon that drives major weather patterns across the globe. While both deep learning (DL), trained on vast climate simulation datasets, including those using the Convolutional Neural Networks (CNN) and 3D-Geoformer, and dynamical models of the North American Multi-Model Ensemble (NMME), based on the physics of the ocean-atmosphere system, have advanced ENSO forecasting independently, each has notable limitations.
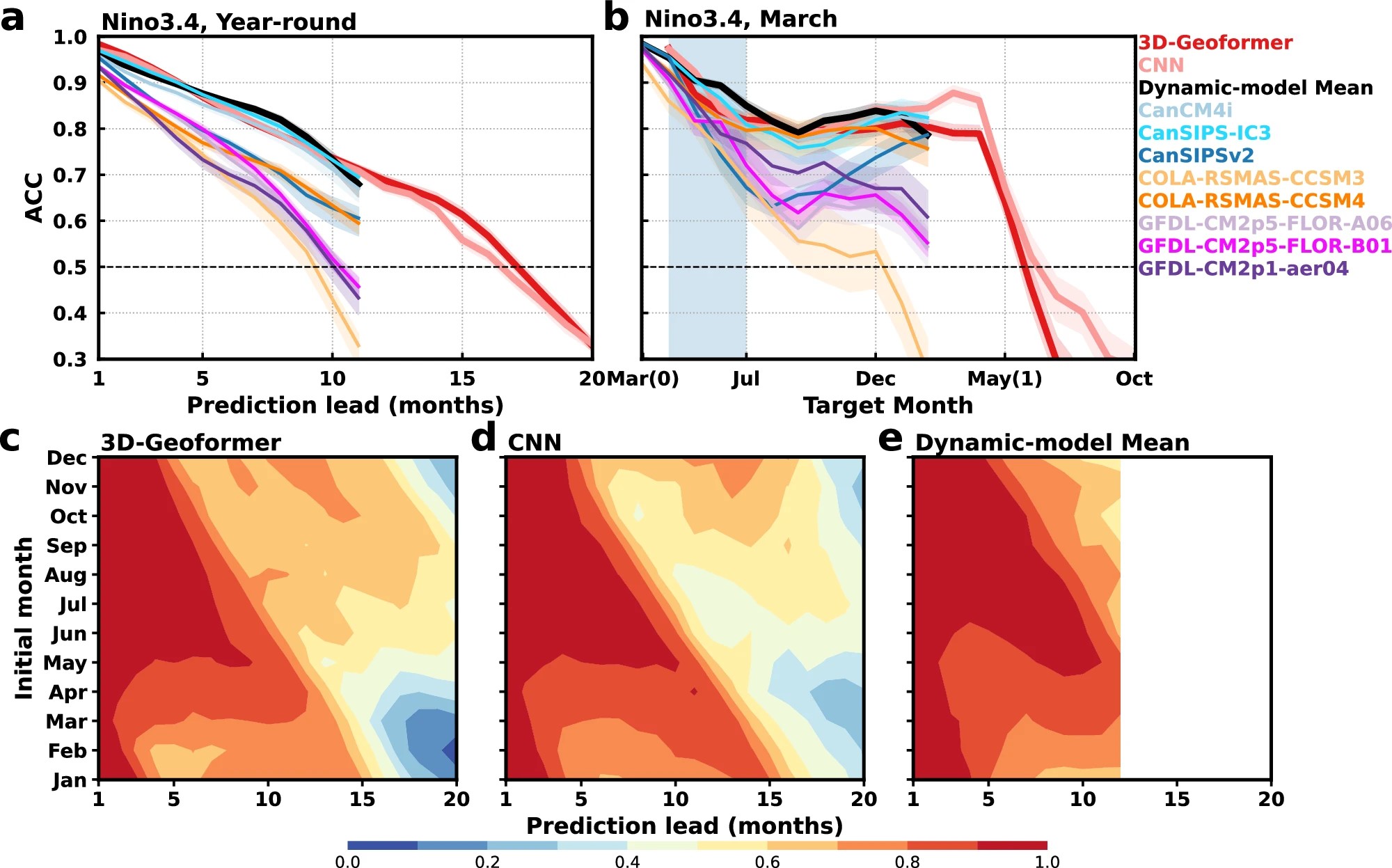
The research team developed two innovative strategies to integrate these approaches.
The first method, Strategy 1, averages forecasts from deep learning models and the multi-model mean of dynamical forecasts. This hybrid approach achieved superior accuracy compared to either method alone, particularly across difficult forecast periods like the boreal spring, when traditional predictability declines.
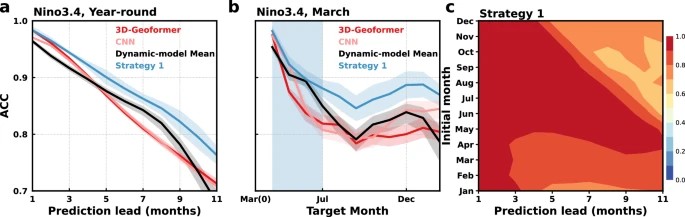
The second method, Strategy 2, uses deep learning predictions as a "first guess" to select more accurate initial conditions for individual dynamical models. This approach significantly improved long-range ENSO predictions, especially beyond eight months, for most models tested.
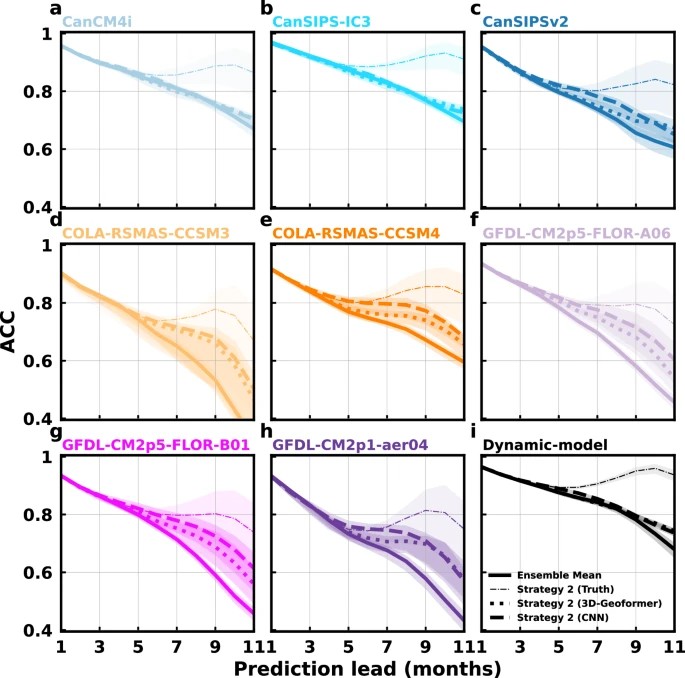
Both strategies demonstrated improved skill in predicting major ENSO events, such as those in 1997–98 and 2015–16, although the deep learning models still underestimated extreme intensities.
While the study marks a significant step forward, the researchers note that operational forecasting using these techniques will require further development. Current deep learning models depend on gridded reanalysis datasets that often lag behind real-world conditions, limiting timely forecasting. Developing deep learning–based climate forecasting models that rely on scattered observational data rather than gridded reanalysis datasets could reduce dependence on update latency, thereby enhancing the timeliness and effectiveness of real-time forecasting and improving their ability to simulate extreme ENSO events.
Enhanced ENSO forecasting has wide-reaching societal and economic benefits. By integrating artificial intelligence into climate science and enhancing early-warning systems for weather and climate extremes, from droughts and floods to disrupted agricultural cycles, models strengthen the boundaries of seasonal forecasting and can improve global preparedness for climate-driven events.
Learn more about "Combined dynamical-deep learning ENSO forecasts."